AI
The Agent-ification of Customer Success: How GenAI is Redefining the CS Function
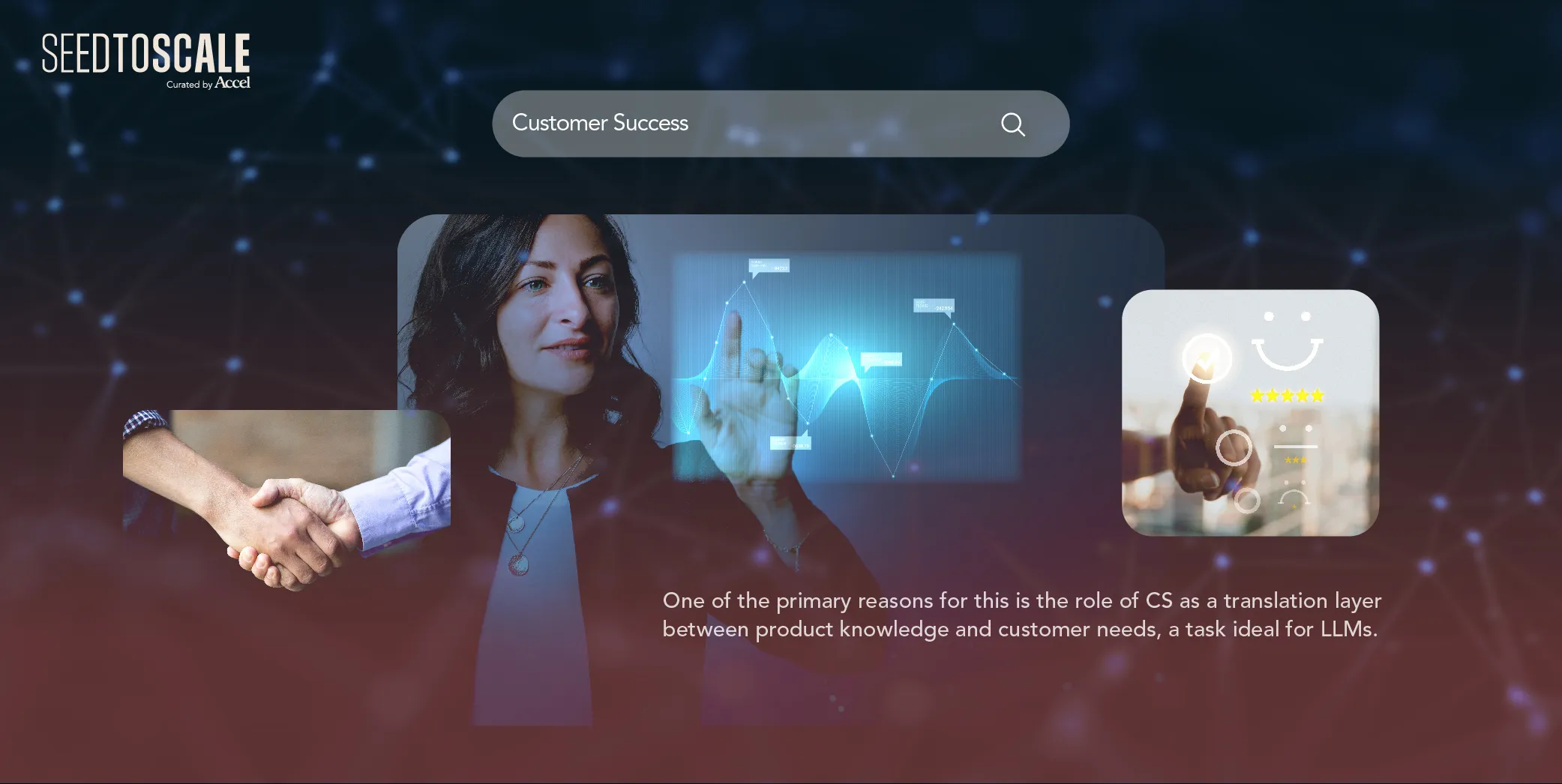
Summary
Customer Success (CS) appears to be one of the few areas of enterprise-readiness for GenAI. Recent developments highlight this, such as Klarna’s impressive results with AI-driven customer support agents and the surge of startups targeting this area, including Brett Taylor’s Sierra.
One of the primary reasons for this is the role of CS as a translation layer between product knowledge and customer needs, a task ideal for LLMs. Solutions Architects translate between customer workflows and product configurations. Success Managers translate between product roadmap and upsell opportunities. Support Agents translate between customer pain points and product documentation. At the risk of oversimplifying, CS’ North Star is its translation quality i.e. the goodness of the fit between the product output and the customer need. And in no part of CS is AI upleveling on translation quality faster than in Support.
Today, LLMs are surprisingly effective at handling basic Support tasks. They are available 24/7, have comprehensive knowledge of product documentation and roadmaps, access all customer interaction histories, and continuously learn from human inputs, whether from customers or product managers. While challenges like hallucinations exist, they seem solvable. Beyond replicating human tasks, AI Support agents can also perform tasks that are difficult for humans, such as summarizing vast numbers of multi-channel customer interactions to help the product team prioritize features, or proactively informing certain customers about feature launches they would care about. Although the focus here is on Support, it’s easy to envision AI expanding into the other more complex CS areas.
So, what does this mean for building an AI-native CS function? First, let’s understand how CS operates today. Except for the highest-tier enterprise customers who receive white-glove service, CS often serves as a patch for missing product features and usability issues, making it a cost center that companies aim to minimize to maintain healthy margins. Without AI, CS leaders address this cost-center challenge by limiting the number of support hours available to SMB customers and charging for overages, increasing the customer-to-CS rep ratio, building online help centers and certifications for customer self-service, and fostering customer communities on platforms like Slack or Discord. While these scaled programs are valuable, they never quite achieve the quality of human help, often leaving customers dissatisfied. This dissatisfaction inevitably leads to firefighting by the CS team, as a dissatisfied customer is a renewal risk, and every renewal is crucial. The choice between a large CS headcount or poor customer satisfaction is now a false dichotomy in the GenAI era we’re entering. For instance, companies can now offer unlimited, high-quality 1:1 Support interactions via AI agents, as Klarna demonstrated. In Success Management, AI agents can take on more complex tasks, such as creating custom dashboards to help customers understand product adoption, recommending features based on usage patterns, and even managing upsell motions all the way to invoicing. In Solution Architecting, AI agents can guide customers through product deployment and configuration as software engineering co-pilots do. Essentially, the promise of AI is allowing companies to adequately serve (and maybe even delight) the next customer with minimal additional cost.
Looking ahead, the key differentiator in CS will no longer just be the talent of the CS team but also the quality of the AI agent(s) managed by the company. The latter is largely a function of the quality of model training data. While anyone can use a foundation model to create a generic upsell deck, having AI that understands the context behind the software purchase, customer usage metrics, and engagement data can create upsell strategies and content that feels personalized and is much more likely to get customer buy-in. Therefore, companies should focus on ensuring that critical customer and product data is recorded and stored according to enterprise best-practices (maintaining a single source of truth for each data source, having clear data provenance etc.). Your data strategy will likely set the ceiling for the quality of your AI strategy.
While all of the above is reasonable conjecture, it’s clear that we’re on a path to AI upleveling Customer Success and I for one have an optimistic outlook: customers will be more satisfied, business margins will improve, humans can focus on strategic work like selling and strategizing, and product teams will receive better insights into customer needs than ever before.
Join our exclusive Bharat community
- Best-in-class Bharat-focused content offering actionable insight for founders and operators
- Specially curated events and thematic summits
We’re looking for the next generation of successful Indian marketplaces. If you’re an early-stage marketplace founder, apply now here or learn more about the #DecodingMarketplaces Startup Hunt.
We’d love to hear about your experiences with marketplaces. Let us share our learnings and build a better and stronger ecosystem. Write to us at seedtoscale@accel.com to be a part of the Accel family.
Subscribe to SeedToScale
/subscribe to get the latest stories from SeedToScale/